Invited Talk1
Speaker: Bo An, Associate Professor, Nanyang Technological University, Singapore

Biography: Bo An is a President’s Council Chair Associate Professor in Computer Science and Engineering, and Co-Director of Artificial Intelligence Research Institute (AI.R) at Nanyang Technological University, Singapore. He received the Ph.D degree in Computer Science from the University of Massachusetts, Amherst. His current research interests include artificial intelligence, multiagent systems, computational game theory, reinforcement learning, and optimization. His research results have been successfully applied to many domains including infrastructure security and e-commerce. He has published over 100 referred papers at AAMAS, IJCAI, AAAI, ICAPS, KDD, UAI, EC, WWW, ICLR, NeurIPS, ICML, JAAMAS, AIJ and ACM/IEEE Transactions. Dr. An was the recipient of the 2010 IFAAMAS Victor Lesser Distinguished Dissertation Award, an Operational Excellence Award from the Commander, First Coast Guard District of the United States, the 2012 INFORMS Daniel H. Wagner Prize for Excellence in Operations Research Practice, and 2018 Nanyang Research Award (Young Investigator). His publications won the Best Innovative Application Paper Award at AAMAS’12, the Innovative Application Award at IAAI’16, and best paper at DAI’20. He was invited to give Early Career Spotlight talk at IJCAI’17. He led the team HogRider which won the 2017 Microsoft Collaborative AI Challenge. He was named to IEEE Intelligent Systems' "AI's 10 to Watch" list for 2018. He is PC Co-Chair of AAMAS’20. He is a member of the editorial board of JAIR and the Associate Editor of JAAMAS, IEEE Intelligent Systems, and ACM TIST. He was elected to the board of directors of IFAAMAS and senior member of AAAI.
Title:When AI Meets Game Theory
Abstract: In January 2017 CMU’s Libratus system beat a team of four top-10 headsup no-limit specialist professionals, which was the first time an AI had beaten top human players in this game. Libratus’s success is purely based on algorithms for solving large scale games and has nothing to do with deep learning! Over the last few years, algorithms for solving large scale games have also been applied to many domains such as security, sustainability, ad-word auction, and e-commerce. For some complex domains with strategic interaction, reinforcement learning is also used to learn an efficient policy. This talk will discuss key techniques behind these success and their applications in domains including games, security, e-commerce, and urban planning.
Invited Talk2
Speaker: Taiki Todo, Assistant Professor, Kyushu University, Japan
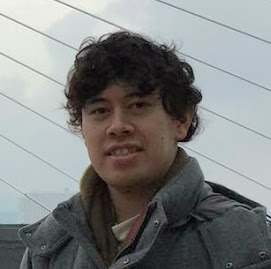
Biography: Taiki Todo is an assistant professor of Graduate School of Information Science and Electrical Engineering (ISEE), Kyushu University. He obtained masters and Ph.D. degrees of Information Science at Kyushu University, in 2010 and 2012, respectively. He has been a JSPS young researcher (2010–2013), a postdoctoral associate in Duke University (2012–2013), a post-doctoral researcher in Kyushu University (2013), an invited professor in Paris-Dauphine University (2016–2017), and a visiting scholar in City University of Hong Kong (2016–2017). His main research field is multi-agent systems, a subfield of artificial intelligence. His research interest lies at the intersection between computer science and game theory, especially mechanism design, i.e., designing incentive mechanisms for various market situations such as auctions, barter exchange, school choice and voting. His research contribution is summarized as mechanism design with uncertainty. Traditionally, the theory of mechanism design have been developed, in the literature of micro-economics, for targeting static environments where the set of agents is fixed and all the information about the agents are observable by the mechanism designer. However, in practice, it is very natural to assume that such a set of agents are not observable apriori and/or some information are uncertain. He therefore focused on various kinds of uncertainty in mechanism design and developed/analyzed several market mechanisms that incentivise agents to behave in an expected/sincere way. He has published several papers from prestigious venues in the field of artificial intelligence, including 3 IJCAI papers (CCF A; CORE A*), 5 AAAI papers (CCF A; CORE A*), 12 AAMAS papers (CCF B; CORE A*), 1 Journal of Artificial Intelligence Research paper (CCF B; SCI), and 1 Fundamenta Informaticae paper (CCF C; SCIExtended). He is the first/corresponding author of 12 papers among them. He has 397 Google Scholar (GS) citations in total, where his h-index is 12 and i10-index is 13 (counted on November 10, 2020). He has been serving as PI for five research grants, three from JSPS (equals to NSFC in China or NSF in US), one from Microsoft Research, and one from a private funding agency. Among them, the largest project grants him 34.5M JPY from Apr 2020 to Mar 2024.
Title:Social Choice with Variable Populations
Abstract: Social choice theory is one of the well-studied mathematical foundations of decision making for multi-agent systems. In the literature of social choice theory, the number of agents in the system is usually assumed to be a constant, and different social choice functions can be applied to different populations. When the number of agents is treated as a variable, e.g., not observable a priori, however, a social choice function must be carefully designed so that it can accept any possible population as input. Indeed, for the open, anonymous, and dynamic environments, the number of agents is not likely observable for the decision maker. In this talk, I will review some traditional models of social choice, introduce possible extensions of them for variable populations, and discuss the relation with mechanism design.